Single-Cell Multi-omics of Circulating Tumor Cells
As oncology analytical approaches are continuously developing, cancer can be understood in deeper and more detailed ways. However, we still struggle to capture potentially important subpopulations of tumor cells and tumor dynamics. Single-cell multi-omics of circulating tumor cells (CTCs) is on its way to becoming the gold standard for tumor surveillance and treatment adjustment within personalized medicine.
Circulating tumor cells (CTCs) are malignant cells shedding off from the tumor into the bloodstream and bear the capability of forming new metastases. Their number is low within the circulation at any given point, but they carry crucial information about the tumor status, and they can be harvested and enriched through liquid biopsy techniques. Liquid biopsy, a minimally invasive technology, has shown its potential for diagnostic use in early cancer detection and continuous monitoring to guide treatment (Seyfried et al.,2013) by extracting and analyzing tumor information within CTCs and circulating tumor DNA (ctDNA). While ctDNA can only be viewed at the genomic level, CTCs can provide information on the transcriptomic, proteomic, and epigenetic planes as well, and these other ‘omics’ analyses show potential for characterizing subpopulations that have an affinity for causing metastasis (Seyfried et al 2013) and can provide a higher resolution view of the clonal evolution and heterogeneity of the main tumor (Visal et al 2022). Here, we will introduce single-cell multi-omics of CTCs, the analytic or experimental integrations of multiple data ‘omics’ modalities in single cells, and show how they can be utilized within the oncological field.
Cancer study techniques have come a long way since the days when only bulk tumor analyses were possible (figure 1). After the first time, when a single cell was (RNA-)sequenced in 2009 (Tang et al 2009), high throughput methods have been developed which can determine the whole genetic content of cells to give cell-level resolution image about the tissue of interest, which can be of use, especially with heterogenous tissue, like tumor tissues. However, information alone about the genetic, transcriptomic, or other omics modality fails to represent cell-to-cell differences between the phenotype and function in their entirety, which means information is needed about epigenetic profiles, spatial distributions, and interactions with the microenvironment, to untangle the tumor’s metastasizing power and its potential to resist medication (Nam et al 2020). Single-cell multi-omics approaches capture the most accurate state of factors that shape the cellular phenotype, and integrating these layers to each other, as well as to bulk data or ctDNA can optimize data extraction to attempt solving the complex relationship between expression, function, and identity (Ortega et al 2017).
Throughout recent years, researchers have been working on ways to integrate all the other omics with cancer genetic data, such as transcriptomics, epigenetics, and proteomics as they are indispensable for a comprehensive understanding of the driving forces of cancer evolution (Nam et al 2020). Nowadays, acquiring data about the entire genome, transcriptome, or other omics is much more affordable as sequencing prices are relatively low and so these methods have become more and more widespread (Li et al 2020). Liquid biopsy is a great addition to the diagnostic palette, as it can provide comparable information about the tumor to tissue biopsies, in the form of CTCs, but in a minimally invasive way (Visal et al 2022). For the analysis of multi-faceted data, improvements in the fields of bioinformatics and data science enable us to reduce inherent noise and integrate the data, which ultimately helps with making sense of the complex nature of single-cell analysis (Ortega et al 2017). For example, Monocle2, an unsupervised algorithm is designed to deconvolute the micro-evolution of cancer cells from single-cell RNA-seq to also approximate their heterogeneity (Trapnell et al 2014).
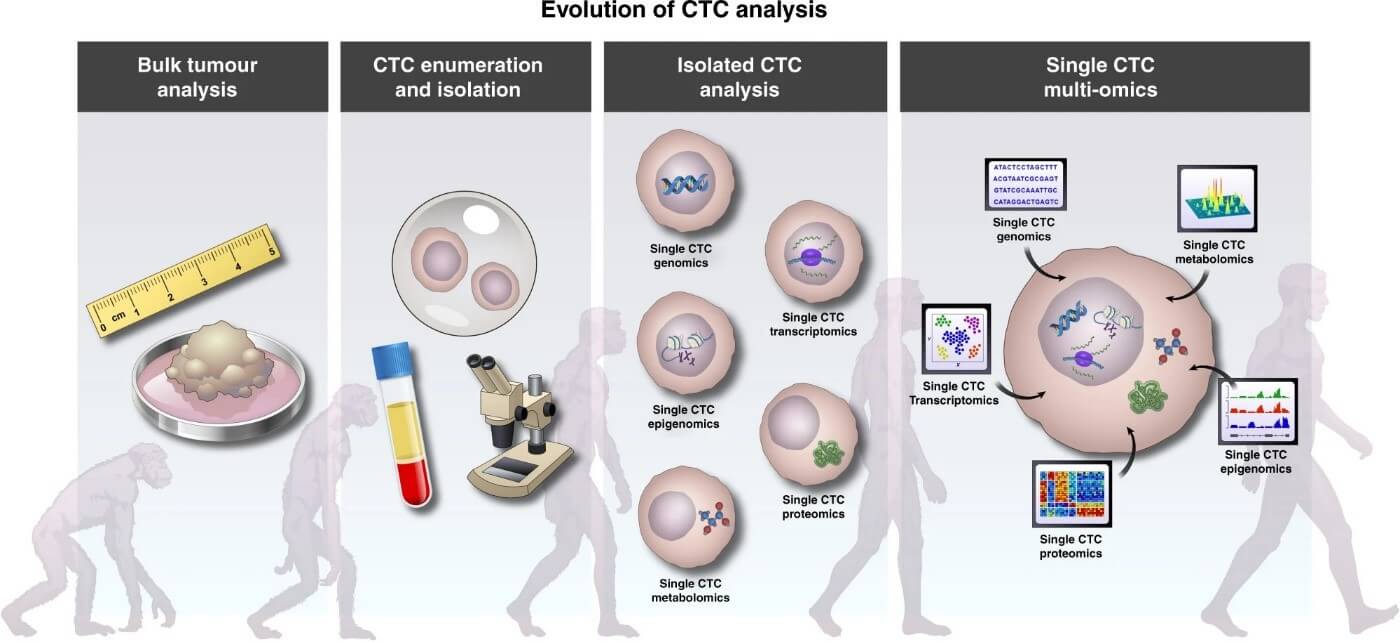
Figure 1: The steps through which single CTC multi-omics developed, as the integration of the separate omics analysis workflows and resulting data mean the future for CTC analysis. (Visal et al 2022)
About the segments of multi-omics
Genomics: The genomic analysis of CTCs has immensely furthered our understanding of intra-tumor heterogeneity through the measurement of mutations (Navin et al 2011), using whole genome sequencing methods. One of these methods is Multiple Displacement Amplification (MDA), which is great at amplifying point mutations as it can reach higher fidelity and coverage than PCR-based methods. The method has been applied to analyze patient-derived CTCs (Wang et al 2014; Lu et al 2020) Even so, challenges remain in the field in reaching high genome coverage and low amplification errors, but the potential for single-CTC analysis remains clear.
Transcriptomics: RNA-Seq, the sequencing of the transcriptome is a good indicator of gene expression as it allows accurate measurement of transcript levels, and it has been successfully implemented for single-cell analysis. However, it has been proving difficult to achieve a full-length sequence from a single cell, and detecting low-abundance transcripts has also been a challenge (Marinov et al 2014). Whole Transcriptome Analysis (WTA) methods, such as Smart-seq (Picelli et al 2014) have improved the number of full-length sequences, and Hydro-seq, a scalable, high throughput single-cell RNA-seq technique, shows capabilities in discovering rare CTC clones crucial to driving cancer progression and metastasis (Cheng et al 2019). So even though limitations exist, single-cell RNA-seq analysis is an important tool for identifying novel biomarkers and rare phenotypes (Visal et al 2022).
Proteomics: Even though RNA-seq approximates the protein content of CTCs, these two profiles do not completely overlap, and proteomics is still a very useful tool for exploring cellular processes such as signal transduction and regulation of transcription, thereby resolving cellular function and phenotype (Visal et al 2022). CTC subpopulations may vastly differ due to genetic diversification and clonal selection, and proteomics holds the key for the characterization of these subgroups, thereby resolving inter- and intra-tumor heterogeneity (Ortega et al 2017). Methods to highlight are Cytometry by Time-of-Flight (CyTOF) techniques which allow for highly quantitative measurement of over 40 proteins in a single cell, and High-Definition Single-Cell Analysis (HD-SCA) which reduces sample enrichment and processing steps of liquid biopsy and has the potential to assess most any cell in a blood sample to simultaneously classify and monitor cancer (Wistuba-Hamprecht et al 2017).
Epigenomics: Epigenomics has an important role in the modulation of gene expression, not dependent on the sequence of DNA, but reversible and dynamic modifications of the DNA molecule. On a single-cell level, investigating the epigenome can identify rare populations and determine the distribution of epigenetic marks between even parent and daughter cells (Clark et al 2016). In the last decade, methods have been developed to analyze several facets of the epigenome at single-cell resolution: DNA methylation (scBS-seq), histone modification (scChIP-seq), DNA accessibility (scATAC-seq) and chromosome conformation (scHiC), which explains the relationship of distant genomic elements through chromatin topology (Clark et al 2016). Within CTCs, the methylation of stem cell genes and miRNAs has been extensively researched, these have been linked to cancer progression and constitute potential targets for treatment (Gkountela et al 2019).
To reiterate: Single-cell multi-omics of CTCs has the potential to transform our knowledge about how cellular function is altered in cancer at an unprecedented resolution, an insight that could be utilized within the fields of personalized medicine.
Recent blogs
Cell-free DNA Fragmentomics: A Promising Predictor of Cancer
In this blog entry, we will explore the recent history, intriguing findings, and tools related to cfDNA fragmentomics.
New developments in the field of circulating tumor cells (2024)
The blog post focuses on how researchers can produce more meaningful, applicable results that directly benefit human health.
Singlera technologies 5: Panseer – detecting pan-cancer signatures years before conventional diagnosis
In the last blog of the year and the concluding chapter of the Singlera series, we are going to explore PanSeer, a blood-based screening test utilizing unique methylation signatures.