Singlera technologies 5: Panseer – detecting pan-cancer signatures years before conventional diagnosis
In the last blog of the year and the concluding chapter of the Singlera series, we are going to explore PanSeer, a blood-based screening test utilizing unique methylation signatures, it redefines early cancer detection by identifying multiple malignancy types years before conventional diagnosis, promising a paradigm shift in oncology research and patient care.
Background
In the landscape of cancer treatment, late-stage diagnoses pose formidable challenges, often limiting effective treatment options and compromising patient outcomes. This critical gap underscores the pressing need for robust early detection mechanisms. However, existing screening tests present limitations, both in efficacy and patient adherence, necessitating the exploration of novel, non-invasive approaches.
In the first blog of the Singlera series, we looked at the immense potential of the methylation haplotype screening technology for early cancer detection from a theoretical point of view, then in blog number 2-4 we highlighted some emerging approaches against major cancer indications. In the present post, the last of the Singlera series, we will shed some light onto an approach which aims to inspect cancer in a more comprehensive way, targeting not a particular disease, but multiple cancer types that might reside in the body, also known as a pan-cancer approach.
PanSeer
Similarly to previous, throughout the blog series discussed applications, PanSeer is a non-invasive liquid biopsy method, investigating cancer methylation markers from circulating tumor DNA (ctDNA) from blood plasma, which is mixed in with cell-free DNA (cfDNA) which is normally present in the bloodstream. PanSeer stands out from other methods because it was validated on the Taizhou Longitudinal Study (TZL), tapping into a cohort of 123,115 initially healthy subjects aged 25 to 90 years.
It provides high sensitivity with its so-called semi-targeted PCR technology, which allows the test to interrogate a high number of regions at high depth and detect spike-ins down to a cancer DNA fraction of 0.1%, which can be crucial for detecting ctDNA with higher accuracy.
The authors in the paper elaborate on how the marker selection process took place. Markers were determined by contrasting the methylation status of cancer tissue of five common cancers and healthy tissue to find regions with significantly different methylation. They identified 477 cancer-specific DMRs that were associated with 657 genes and 10,613 CpG sites. This large number of regions was fed into a machine learning algorithm as features to build a classification model which can discriminate disease and healthy samples with the highest certainty. For this step, the authors chose cfDNA samples from a large amount of kept samples from the Taizhou Hospital Biobanks, which were taken as part of the Taizhou Longitudinal Study (TZL). This way, they were able to train the classifier also on “pre-diagnosis” samples, which is cfDNA from patients who were diagnosed with cancer only years after the blood draw.
Chen et al utilized 207 healthy, 110 post-diagnosis samples, and 93 pre-diagnosis samples for the training set and 113 post-diagnosis and 207 healthy samples for the first testing phase. Here, they established that the classifier accurately detects post-diagnosis samples, with 88% sensitivity and 96% specificity. Afterwards, they used samples from 143 apparently healthy patients that were later diagnosed with cancer within 4 years. For this cohort, the authors observed 95% sensitivity where the specificity ranged from 91% to 100% among different cancer types.
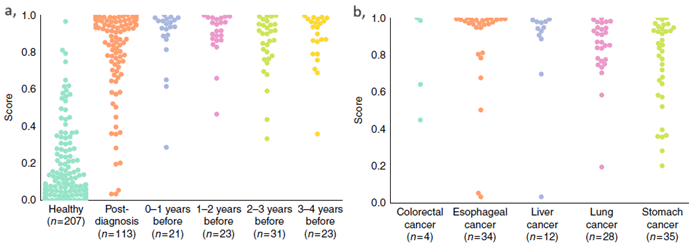
Figure a-b: Sample classification is based on scores provided by the machine learning algorithm, the larger the separation between healthy and diseased, the better. a, PanSeer scores separated by healthy/post-diagnosis/pre-diagnosis. b, PanSeer scores of post-diagnosis samples separated by cancer type.
These tests elucidate PanSeer’s capabilities which rest on the achievements of the Taizhou Longitudinal Study. Over the course of the study’s seven years, blood from 123,115 healthy patients was taken to store and the patients were dynamically followed-up in regular intervals. This way, 575 individuals were diagnosed with stomach, esophagus, colorectal, lung, or liver cancer within four years of the initial blood draw. Through retrospective analysis, PanSeer remarkably identified cancer in pre-diagnosis samples, substantiating its prowess by showing that cancer can be non-invasively detected up to four years before the current standard of care.
Limitations
Further extensive longitudinal studies are needed to solidify the test’s efficacy. Also, pan-cancer approaches inherently pose challenges stemming from the problem of having to detect the origin of the cancer signal. Without figuring out the cancer’s tissue of origin, the patient must go through several tiresome inspections of the common cancer types which may not even give a definitive answer. However, liquid biopsy based cancer origin detecting methods are evolving and getting more accurate.
Conclusion
After bridging some of these limitations, pan-cancer liquid biopsy tests are most likely going to reshape oncological practices and make a huge difference in preventing and curing cancer in the future, considering such applications are advancing at a rapid pace. PanSeer is one of these applications, leveraging a comprehensive set of markers derived from methylation signatures, demonstrating high accuracy in identifying multiple cancer types years before conventional screening methods.
Recent blogs
New developments in the field of circulating tumor cells (2024)
The blog post focuses on how researchers can produce more meaningful, applicable results that directly benefit human health.
Singlera Technologies 4: Catching Pancreatic Cancer early with intricate methylation markers
Enter PDACatch, a novel assay developed to detect circulating tumor DNA methylation signatures with greater sensitivity and accuracy.
Singlera technologies 3: Methylation signatures for hepatocellular carcinoma detection
Identification of DNA methylation signatures for hepatocellular carcinoma detection and microvascular invasion prediction.