Singlera technologies 1: Methylation haplotyping
Identification of methylation haplotype blocks aids in the deconvolution of heterogeneous tissue samples and tumor tissue-of-origin mapping from plasma DNA. – Guo et al.
Highlights
Methylation haplotyping is a promising strategy for the early detection of cancer and its primary growth site from a non-invasive liquid biopsy and it can also be used for monitoring tumor progression and metastasis to multiple organs. This method improves the robustness and sensitivity of previous DNA methylation-based cancer detection techniques by determining methylation haplotype blocks (MHBs) and establishing a novel metric called methylation haplotype load (MHL).
Introduction
It has been known for decades that cancer alters its methylation status drastically compared to the healthy tissue from which it originates (Jones et al.). The advantage of studying methylation (i.e., epigenetics) as opposed to nucleotide changes (i.e., genetics) is that it is more widespread throughout the genome and leaves more marks indicative of cancerous processes.
In patients with cancer, plasma contains circulating cell-free DNA (cfDNA) fragments from both normal and malignant cell types. To determine fragments with cancer origin, two approaches have been utilized mainly: individual CpG methylation fractions (IMFs), where many CpG states are independently considered, and average methylation fraction (AMF), where the methylation state of a region is averaged. In both approaches, the values of thousands of positions/regions are combined into a classifier which outputs a classification score about the probability of disease. Most of these recent efforts are fundamentally limited by the technical noise and moderate sensitivity as they rely on the methylation level of individual CpG sites (Sharma et al.).
This is where Methylation Haplotype Blocks (MHB) come into the picture. MHBs are regions of the human genome in which adjacent CpG sites show coordinated methylation. These commonly occurring regions exist because of the phenomenon of enzyme processivity (after initiation, the enzyme methylates or demethylates multiple sites in a row). This definition was created by an extension of genetic linkage disequilibrium, based on the same phenomenon just for genetic information (Shoemaker et al.) and Pearson correlation to measure the degree of coupled CpG methylation within short, CpG-rich regions (Guo et al., visual representation Figure 1). The superior sensitivity of multi-CpG haplotypes in detecting tissue-specific signatures in cfDNA has already been demonstrated by Lehmann-Werman et al., however, only on Illumina 450k methylation arrays, which show low genomic coverage. The authors of the paper above have instead searched for tissue-specific MHBs across the full genome and proved to improve the robustness and accuracy of prediction from DNA methylation analysis while capturing even low-frequency alleles within the sparse coverage of cfDNA.
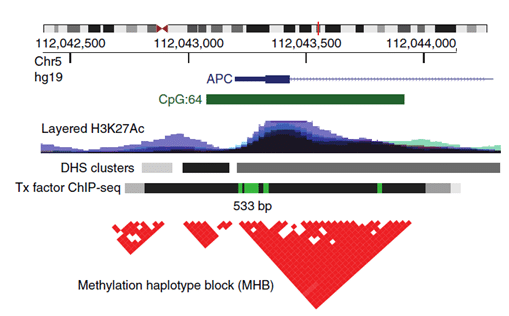
Figure 1.: An example of an MHB at the promoter of the gene APC. Tx, transcription; DHS, DNase-I-hypersensitive sites. From: Guo et al.
Results
First, the authors identified all MHBs in the genome with their definition of coordinated methylation using whole-genome bisulfite sequencing (WGBS) data of 61 samples, which resulted in 147888 MHBs with a minimum of three CpGs per block at an average size of 95 base pairs. This is importantly smaller than the average size of cfDNA fragments of 150 base pairs (Dennis Lo et al.). Local CpG density was not an aspect that justified selection alone. Within healthy samples, almost all CpGs of these MHBs were perfectly coupled (r^2 ~ 1), within cancer samples less so, however, the majority of MHBs in cancers still contained tightly coupled CpGs (87.8%).
Next, haplotyping was demonstrated as a good determinant of a tumor’s tissue of origin. Unsupervised clustering with the 15% of most variable MHBs showed that, regardless of the data sources, samples of the same tissue origin are grouped together. As tissue specificity proved to be a stronger signal than cancer-specific methylation, they continued with a joint analysis of the cancer signature and the tissue-of-origin signature, as it seemed to be more sensitive than focusing on the cancer signature alone.
Crucially, the authors proposed a block-level metric, termed methylated haplotype load (MHL), which is the fraction of fully methylated haplotypes at different lengths, so it measures how much neighboring CpGs are methylated in tandem (shown in Figure 2). To test whether MHL was an informative metric, the performance between MHL and preceding methods, AMF, and IMF was compared, using the previously predicted MHBs. It was demonstrated that MHL exhibits a better signal-to-noise ratio than the AMF and IMF for sample clustering. IMF performed the worst of the three, which might be due to the higher biological or technical variability of individual CpG sites. Coordinated methylation was also capable of distinguishing blocks with the same average methylation levels.
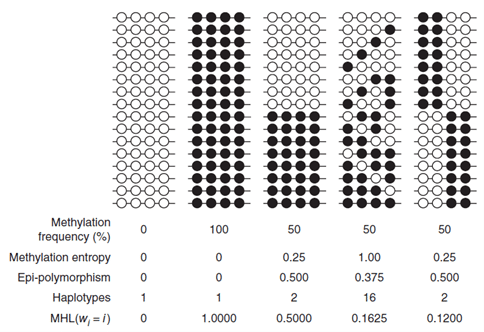
Figure 2.: Toy cases of differences in coordinated methylation which show how the MHL values distinguish them when other metrics are less informative. From: Guo et al.
MHL calculation has been utilized during the biomarker selection process for colorectal cancer (CRC) and lung cancer. The authors looked for MHBs from cancer tissue which do not appear in matched healthy tissue also with low MHL in plasma. Thereby they identified cancer-associated highly methylated haplotypes (caHMHs). Such haplotypes were present only in the tumor tissues and the matched plasma from the same patient, but not in whole blood or any other non-cancer samples. 81 and 94 caHMHs were determined for CRC and lung cancer samples, respectively, and some of these regions (such as HOXA3) were aberrantly methylated in both cancer types. The capabilities of the MHL values of caHMHs for cancer detection have been compared to AMF’s, and IMF’s and it showed clear improvements in the area under the curve (AUC) metric for both cancers.
Summary
Methylation haplotyping is a promising technique utilizing the power of whole-genome sequencing for cancer detection from a non-invasive blood draw. It’s improving early cancer detection sensitivity and specificity of previous methylation applications while providing a framework for establishing tissue-specific cancer methylation markers.
Recent blogs
A New Era in Liver Cancer Detection: The Promise of HepaAiQ
In this blog entry, we will explore the recent history, intriguing findings, and tools related to cfDNA fragmentomics.
Cell-free DNA Fragmentomics: A Promising Predictor of Cancer
In this blog entry, we will explore the recent history, intriguing findings, and tools related to cfDNA fragmentomics.
New developments in the field of circulating tumor cells (2024)
The blog post focuses on how researchers can produce more meaningful, applicable results that directly benefit human health.